Prediction of heat load
alitiq’s precise heat load forecasts enable you as a heat supplier to know today how much heat will be required in your heating network tomorrow and in the following days.
Do you want to maximise your energy revenue? Do you want to optimise the operation of your power plant portfolio?
The expected heat demand must be optimally predicted so that you can optimise your power plant schedule for the expected revenues. The forecasts currently available on the market are highly uncertain, can result in high balancing energy costs and increase your business risk.
With forecasts from alitiq, you can reduce your individual revenue risk!
Your benefits
1
Saving CO2 and generation costs
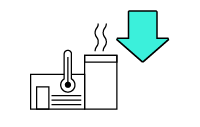
For every 1,000 households, you save more than 100 tonnes of CO2 and 700 MWh of gas per year
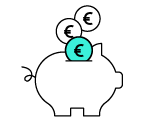
Savings of up to €100,000 per year per 1000 households
2
Highest forecasting quality through the use of AI and multi-model methods
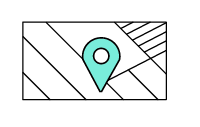
Customised forecast for your supply area
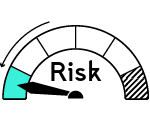
Significant reduction of your risk
3
Simple and fast provisioning
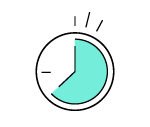
20 hours less personnel expenditure per month
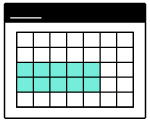
Very rapid onboarding
Forecast improvement
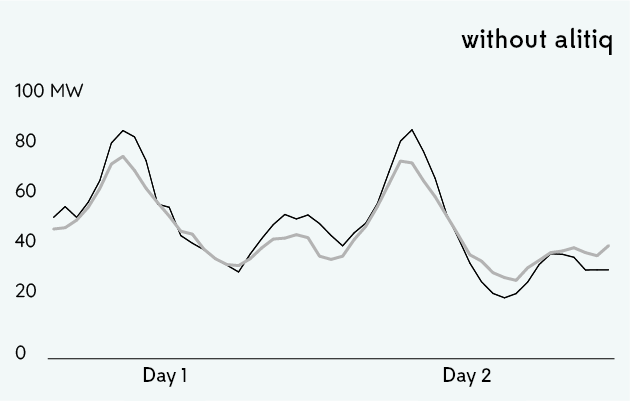
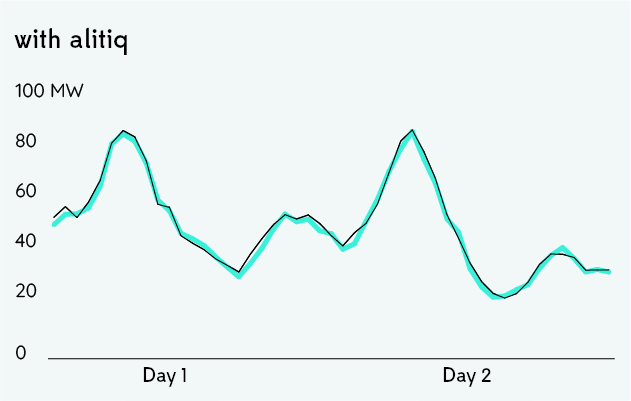

The alitiq engine forecasts the heat load to the hour.
Forecasts from alitiq are up to 40 per cent more accurate than the market standard.
alitiq provides the forecasts several times a day according to your requirements.
It's so easy to get the forecasts to you
Step 1
Initial data exchange
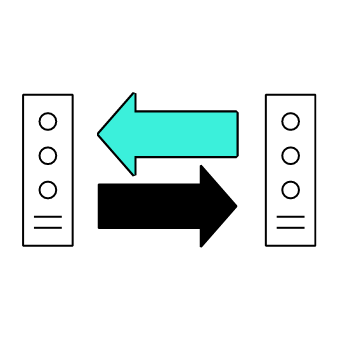
Step 2
Calculation of your individual model with the alitiq engine
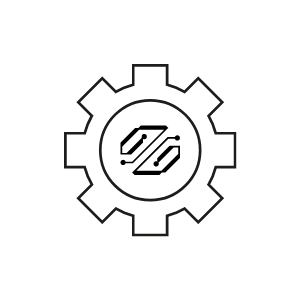
Step 3
Provision of your forecasts via Web API
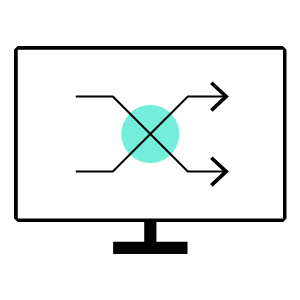
You are interested?
Customer voice
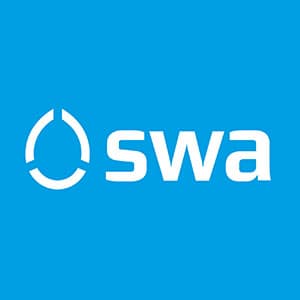
Stadtwerke Augsburg
You are interested?
Arrange a non-binding consultation with your personal contact Matthias Habel immediately.
Do you need other energy forecasts? The alitiq engine can work with any target values. That’s why we can also forecast your process individually!
Talk to our forecasting experts!
About alitiq
Extensive experience in meteorology and data science enables us to calculate the influence of the weather on individual business processes. This enables us to make the process itself predictable.